Professional Education
Abiomed is committed to providing information useful to healthcare professionals, including clinical medical activities and product information. This website provides educational content for healthcare professionals covering a wide range of topics, including summaries of academic conferences and publications related to Impella, patient management, and troubleshooting for complications.
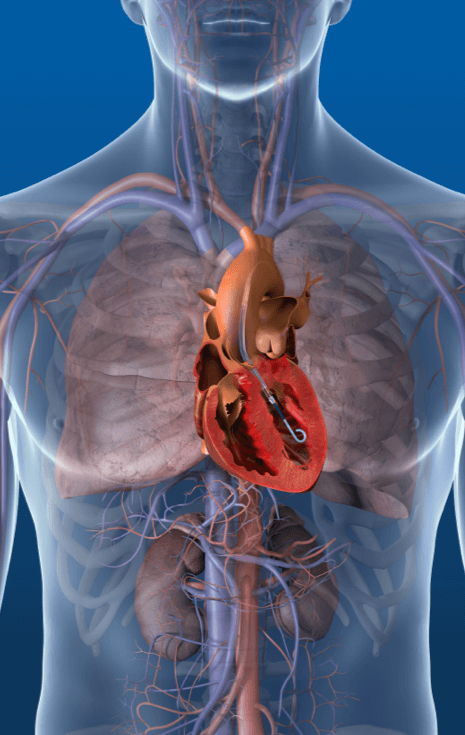